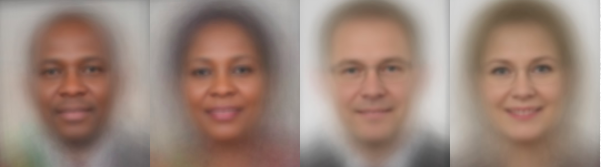
The Gender Shades project evaluates the accuracy of AI powered gender classification products.
This evaluation focuses on gender classification as a motivating example to show the need for increased transparency in the performance of any AI products and services that focused on human subjects. Bias in this context is defined as having practical differences in gender classification error rates between groups.
1270 images were chosen to create a benchmark for this gender classification performance test.
The subjects were selected from 3 African countries and 3 European countries. The subjects were then grouped by gender, skin type, and the intersection of gender and skin type.
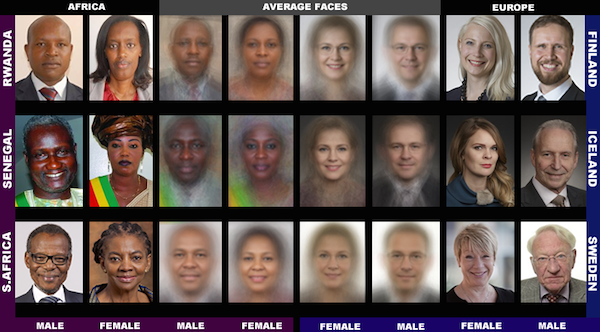
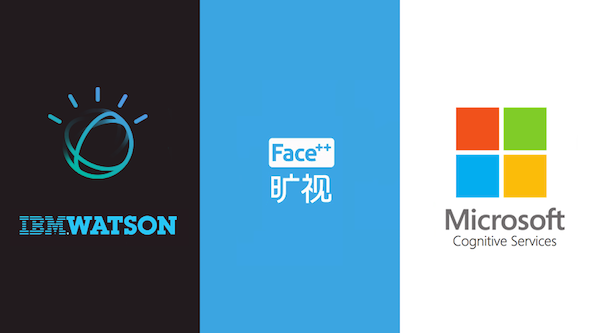
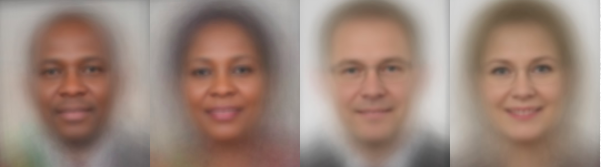
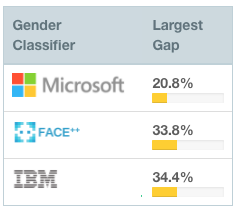
IBM Watson leaders responded within a day after receiving the performance results and are reportedly making changes to the Watson Visual Recognition API. Official Statement.
An internal evaluation of the Azure Face API is reportedly being conducted by Microsoft. Official Statement. Statement to Lead Researcher.
Face++ has yet to respond to the research results which were sent to all companies on Dec 22 ,2017
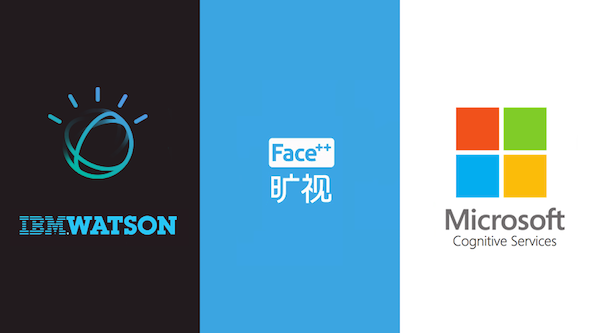
Inclusive product testing and reporting are necessary if the industry is to create systems that work well for all of humanity. However, accuracy is not the only issue. Flawless facial analysis technology can be abused in the hands of authoritarian governments, personal adversaries, and predatory companies. Ongoing oversight and context limitations are needed.
For interested readers, authors Cathy O'Neil and Virginia Eubanks explore the real-world impact of algorithmic bias.
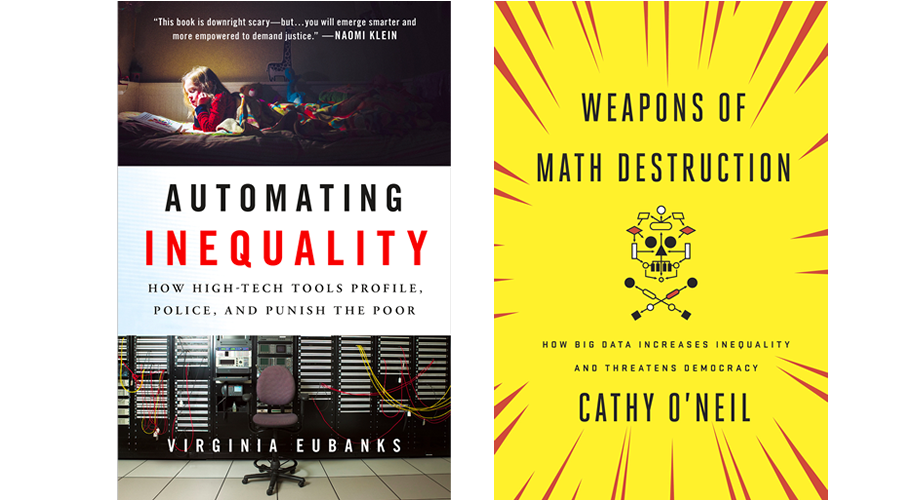
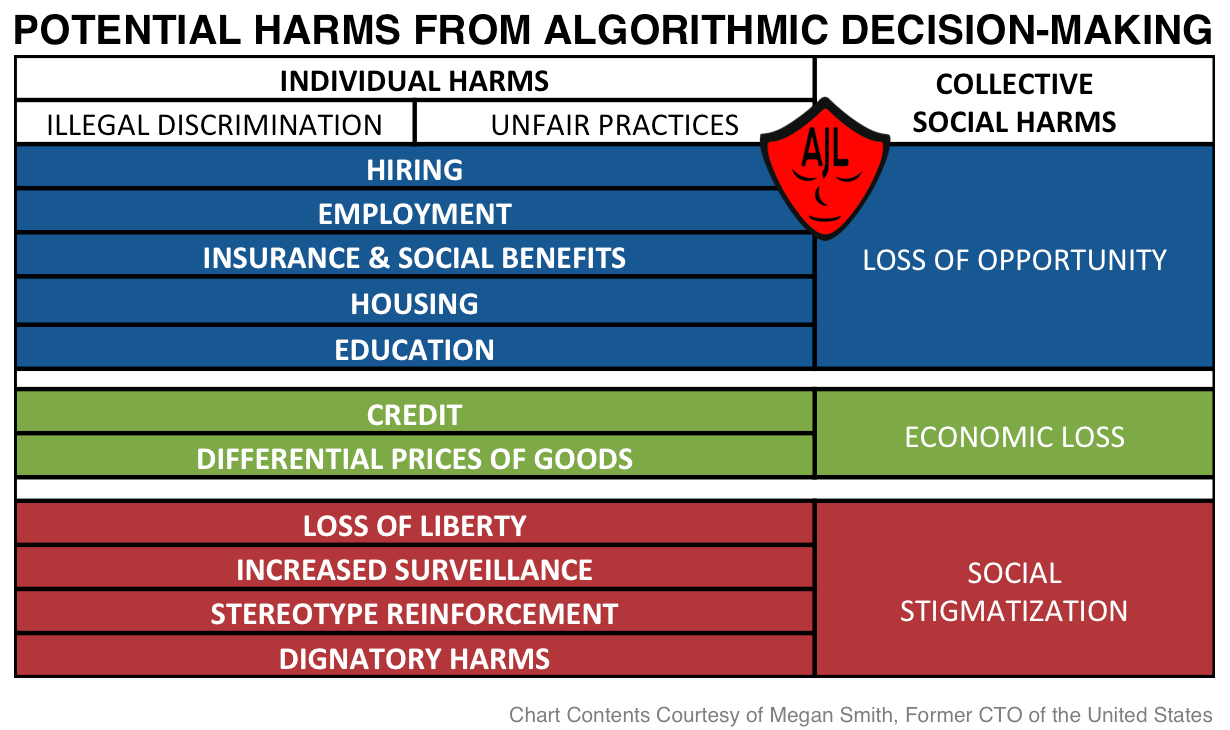
We risk losing the gains made with the civil rights movement and women's movement under the false assumption of machine neutrality. We must demand increased transparency and accountability.
Dive Deeper:
Gender Shades Academic Paper
Test Inclusively:
Request external performance test
Request Pilot Parliaments Benchmark
Gender Classifier | Overall Accuracy on all Subjects in Pilot Parlaiments Benchmark (2017) |
---|---|
![]() |
93.7%
|
![]() |
90.0%
|
![]() |
87.9%
|
Gender Classifier | Female Subjects Accuracy | Male Subjects Accuracy | Error Rate Diff. |
---|---|---|---|
![]() |
89.3%
|
97.4%
|
8.1%
|
![]() |
78.7%
|
99.3%
|
20.6%
|
![]() |
79.7%
|
94.4%
|
14.7%
|
Gender Classifier | Darker Subjects Accuracy | Lighter Subjects Accuracy | Error Rate Diff. |
---|---|---|---|
![]() |
87.1%
|
99.3%
|
12.2%
|
![]() |
83.5%
|
95.3%
|
11.8%
|
![]() |
77.6%
|
96.8%
|
19.2%
|
Gender Classifier | Darker Male | Darker Female | Lighter Male | Lighter Female | Largest Gap |
---|---|---|---|---|---|
![]() |
94.0%
|
79.2%
|
100%
|
98.3%
|
20.8%
|
![]() |
99.3%
|
65.5%
|
99.2%
|
94.0%
|
33.8%
|
![]() |
88.0%
|
65.3%
|
99.7%
|
92.9%
|
34.4%
|